In an increasingly digital world, artificial intelligence (AI) is becoming more widespread. AI is used to automate a variety of tasks, from data analysis to customer service. But with the rise of AI comes the need for transparency and accountability. This is where MLOps and explainability come into play.
MLOps, or machine learning operations, is a process for managing the development, deployment, and maintenance of machine learning models. Explainability helps to ensure that decisions made by AI systems are transparent and accountable by providing insights into how they work. In this blog post, we’ll explore the importance of explainability in machine learning and how MLOps can help ensure transparency and accountability in AI systems.
Why Explainability Matters?
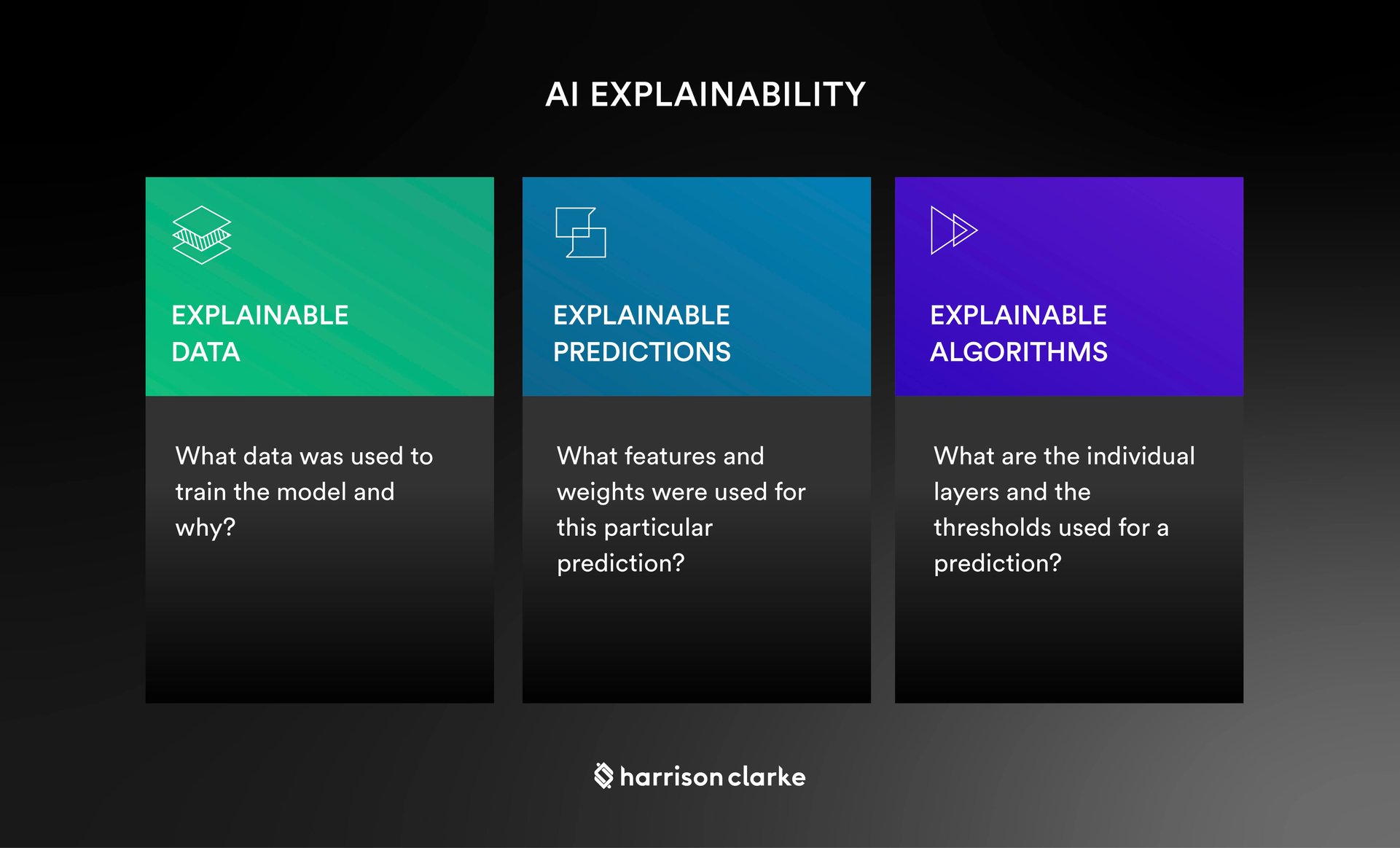
Explainability is important because it provides users with insight into how an AI system works and why it makes certain decisions. It also gives developers a better understanding of their models so that they can identify any issues or potential areas for improvement. Additionally, explainability can help to increase user trust in an AI system by providing transparency about its decisions. Finally, explainability can be used to identify any potential ethical or legal issues with an AI system's decision-making process.
Techniques for Explainability
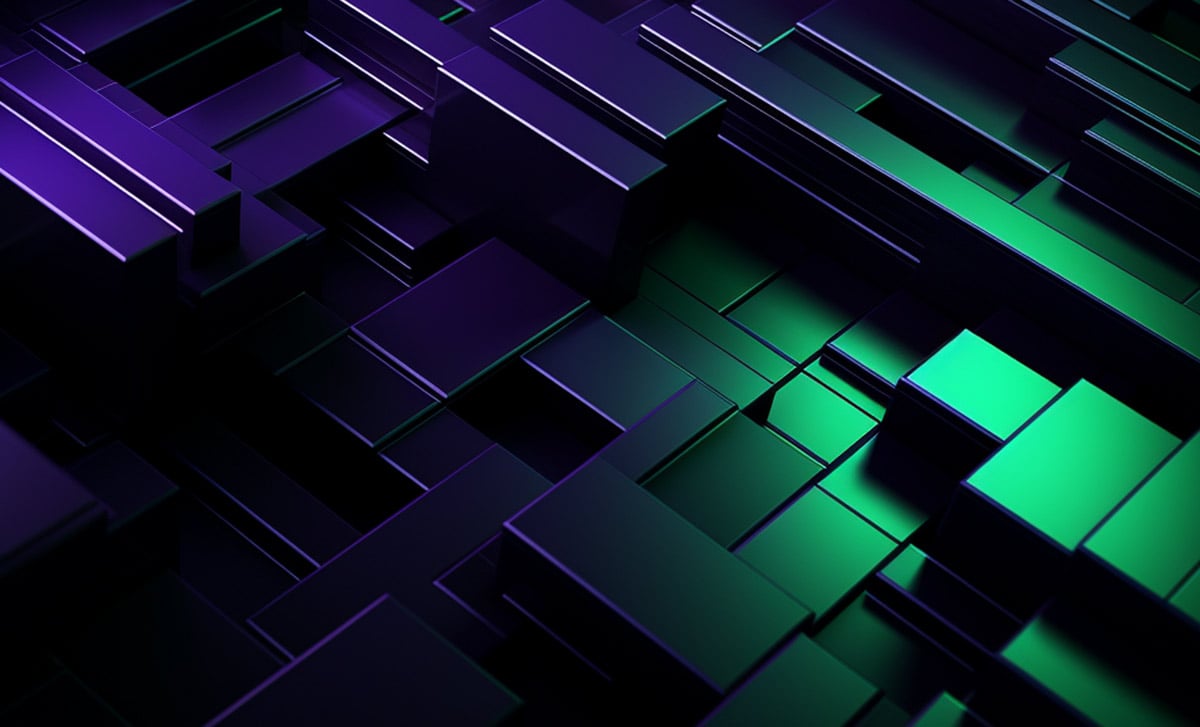
Explainable AI typically involves two main techniques – feature importance and model interpretation. Feature importance measures which features have the greatest impact on a model’s predictions, while model interpretation provides an understanding of how different features interact with each other to influence a model’s output. These techniques can help developers gain insights into their models so that they can make adjustments as needed or deploy new versions as needed. Additionally, they can help users understand why an AI system has made certain decisions so that they can trust the system’s results.
Real-World Examples of Explainable AI
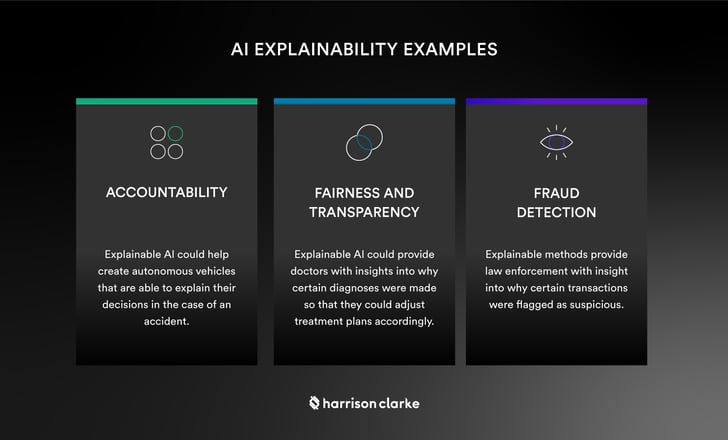
Explainable AI has been used in a variety of real-world applications ranging from medical diagnostics to fraud detection systems. For example, in medical diagnostics, explainable systems have been used to provide doctors with insights into why certain diagnoses were made so that they could adjust treatment plans accordingly. Similarly, fraud detection systems have used explainable methods to provide law enforcement with insight into why certain transactions were flagged as suspicious so that they could investigate further if necessary. These examples demonstrate the power of explainable AI when it comes to ensuring transparency and accountability in decision-making processes powered by artificial intelligence.
Explainability is essential for ensuring transparency and accountability in artificial intelligence systems. By providing insights into how an AI system works and why it makes certain decisions, explainable technologies help to increase user trust while helping developers identify potential areas for improvement or ethical/legal issues with their models’ decision making processes. MLOps helps ensure these benefits by providing a process for managing the development, deployment, and maintenance of machine learning models over time – making sure that any changes implemented are well documented along the way – ultimately helping organizations stay compliant while improving user experience at every turn!